LORE Stable and Actionable
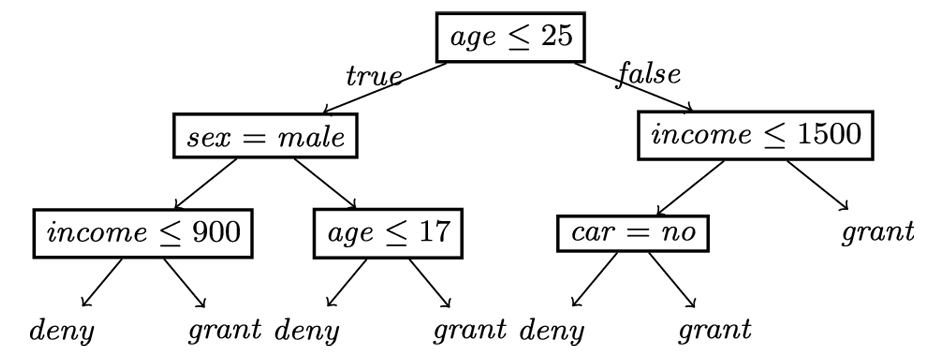
Recent years have witnessed the rise of accurate but obscure classification models that hide the logic of their internal decision processes. Explaining the decision taken by a black-box classifier on a specific input instance is therefore of striking interest. We propose a local rule-based model-agnostic explanation method providing stable and actionable explanations (Guidotti et al., 2019; Guidotti et al., 2022). An explanation consists of a factual logic rule, stating the reasons for the black-box decision, and a set of actionable counterfactual logic rules, proactively suggesting the changes in the instance that lead to a different outcome. Explanations are computed from a decision tree that mimics the behavior of the black-box locally to the instance to explain. The decision tree is obtained through a bagging-like approach that favors stability and fidelity: first, an ensemble of decision trees is learned from neighborhoods of the instance under investigation; then, the ensemble is merged into a single decision tree. Neighbor instances are synthetically generated through a genetic algorithm whose fitness function is driven by the black-box behavior. Experiments show that the proposed method advances the state-of-the-art towards a comprehensive approach that successfully covers stability and actionability of factual and counterfactual explanations.