Ethics and Legal
In this direction, the work of Line 5 has started the investigation of the interplay between explainability and privacy, and explainability and fairness under different viewpoints. In particular, the research of this line tries to answer the following questions:
Can explanation methods, introducing a level of transparency in the whole decision process, might jeopardize individual privacy of people represented in the training data? Can explanation methods help in understanding the reason for possible ethical risks associated with the use of AI systems (e.g., privacy violations and biased behaviors)? Can explanation methods be fundamental for discovering other ethical issues like unfair behavior of AI systems? Starting from these questions, we have scientifically contributed with the following studies. Concerning the ability of explanation methods to explain some ethical risks such as privacy and biases, in [NPM2020,NPN2020] we propose EXPERT, an EXplainable Privacy ExposuRe predicTion framework. It is a tool that exploits Explainability as a tool for increasing privacy user awareness. We applied EXPERT on the privacy risk prediction and explanation of both tabular data [NPM2020] and sequential data [NPN2020]. In the first setting we considered the mobility context where for each user we have the historical movements (a spatio-temporal trajectory). In this context, EXPERT using the privacy risk exposure module extracts from human mobility data an individual mobility profile describing the mobility behavior of any user. Second, for each user it simulates a privacy attack and quantifies the associated privacy risk. Third, it uses the mobility profiles of the users with their associated privacy risks to train a ML model. For a new user, along with the prediction of risk, EXPERT also provides an explanation of the predicted risk generated by the risk explanation module. EXPERT exploits two state-of-the-art explanation techniques, i.e., SHAP and LORE [GMG2019]. In the second setting [NPN2020] the prediction phase is not based on a mobility profile but on the trajectory itself and given the absence of features we used only SHAP as an explanation method. In [PPB2021] we also present FairLens, a framework able to detect and explain potential bias issues in Clinical Decision Support Systems (DSS). FairLens allows testing the clinical DSS before its deployment, i.e., before handling it to final decision-makers such as physicians and nurses. FairLens takes bias analysis a step further by explaining the reasons behind the poor model performance on specific groups; in particular, it uses GlocalX to explain which elements in the patients’ clinical histories are influencing the misclassification.
Concerning the use of explainability as a means for discovering unfair behaviors, in [MG2021], we propose FairShades, a model-agnostic approach for auditing the outcomes of abusive language detection systems. FairShades combines explainability and fairness evaluation within a proactive pipeline to identify unintended biases and sensitive categories toward which the black box model under assessment is most discriminative. It is a task-specific approach for abusive language detection: it can be used to test the fairness of any abusive language detection system working on any textual dataset. However, its ideal application is on sentences containing protected identities, i.e., expressions referring to nationality, gender, etc., as the primary scope is to uncover biases and not explain the reasons for the prediction.
Research line people
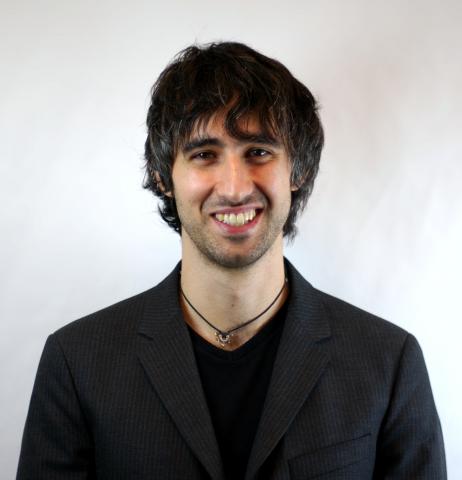
Guidotti
Assitant Professor
University of Pisa
R.LINE 1 ▪ 3 ▪ 4 ▪ 5
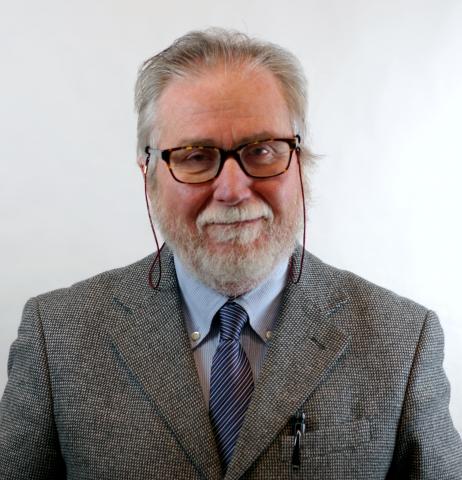
Turini
Full Professor
University of Pisa
R.LINE 1 ▪ 2 ▪ 5
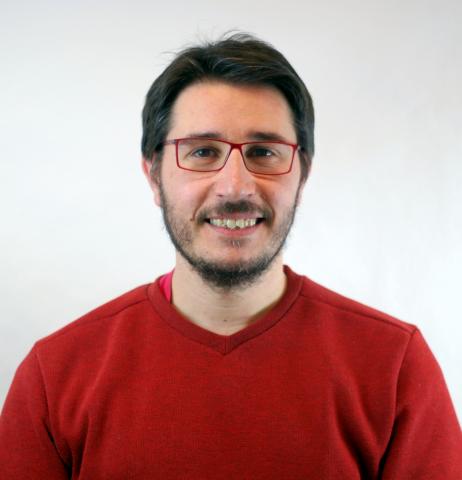
Rinzivillo
Researcher
ISTI - CNR Pisa
R.LINE 1 ▪ 3 ▪ 4 ▪ 5
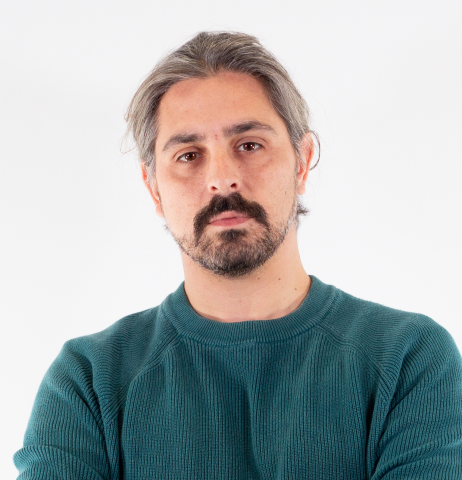
Beretta
Researcher
ISTI - CNR Pisa
R.LINE 1 ▪ 4 ▪ 5
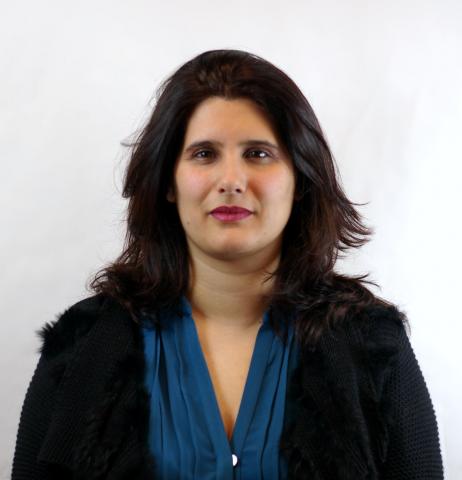
Monreale
Associate Professor
University of Pisa
R.LINE 1 ▪ 4 ▪ 5
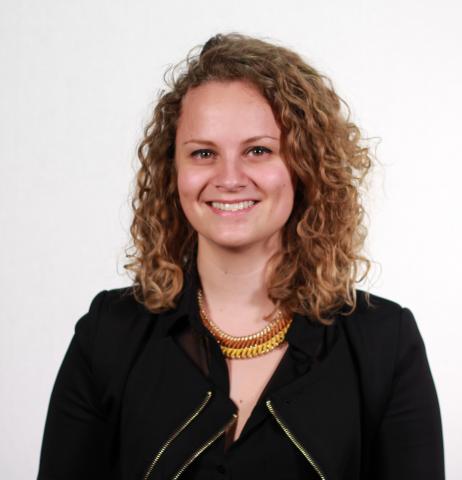
Panigutti
Phd Student
Scuola Normale
R.LINE 1 ▪ 4 ▪ 5
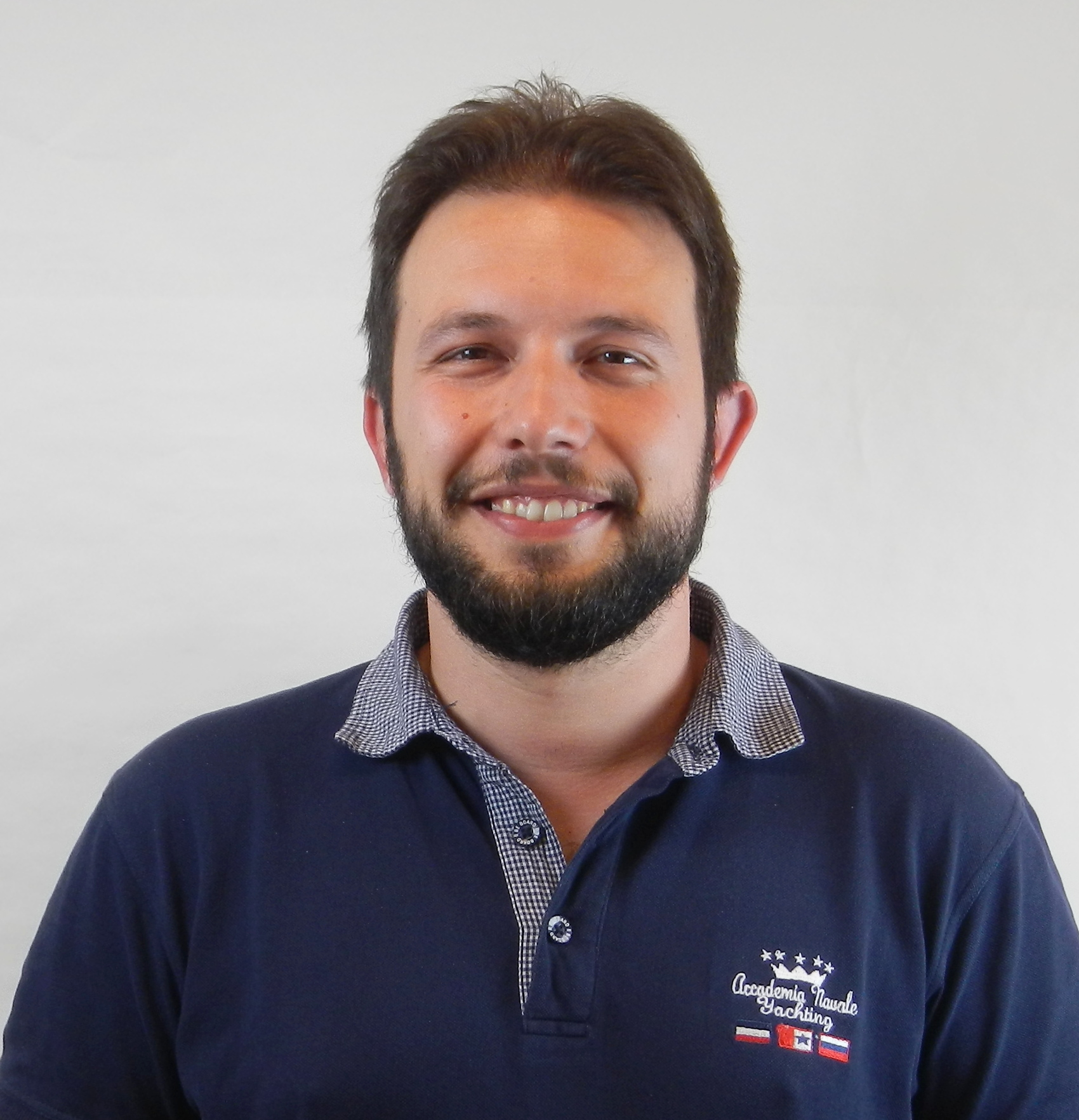
Pellungrini
Researcher
University of Pisa
R.LINE 5
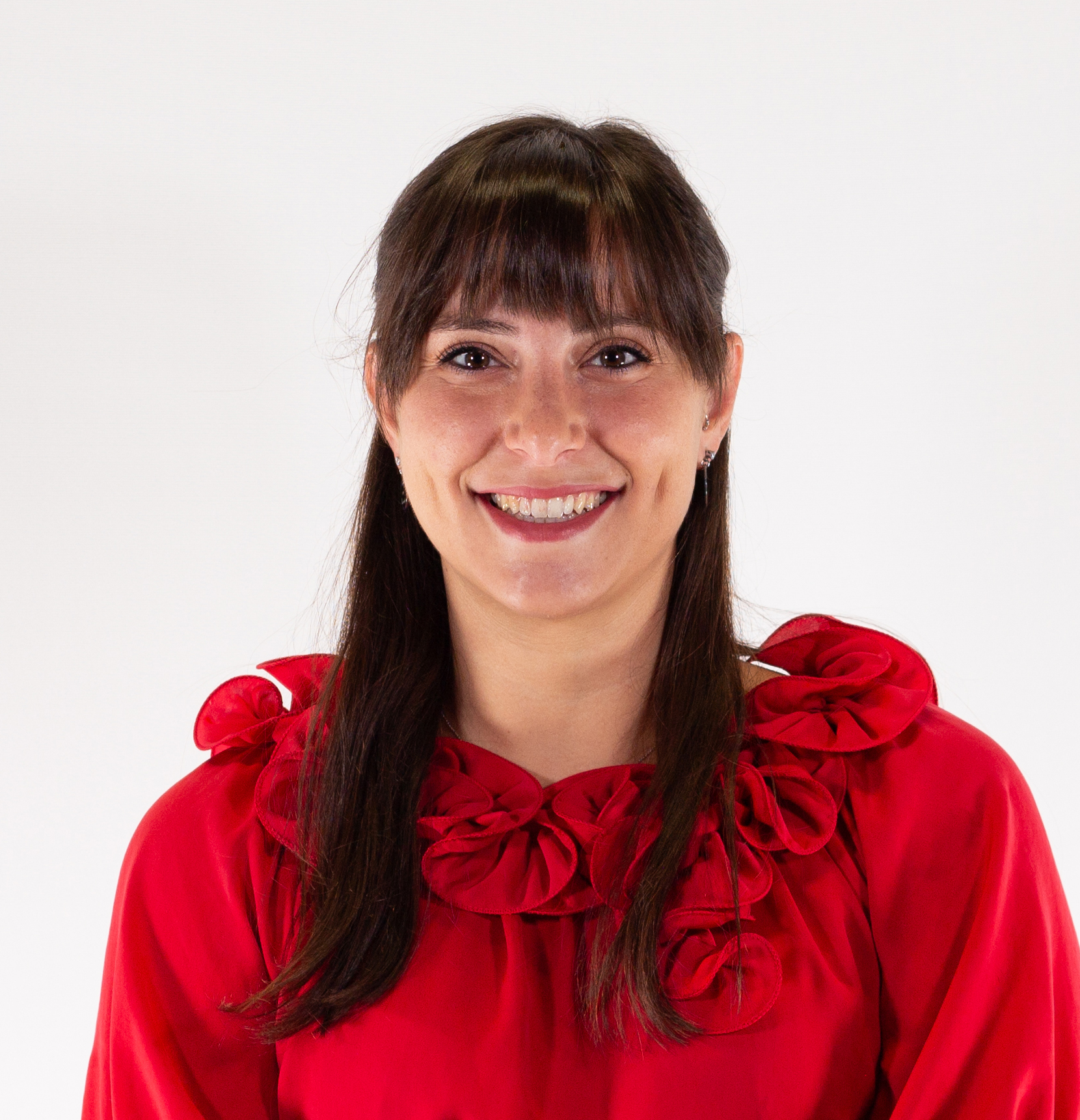
Naretto
Post Doctoral Researcher
Scuola Normale
R.LINE 1 ▪ 3 ▪ 4 ▪ 5
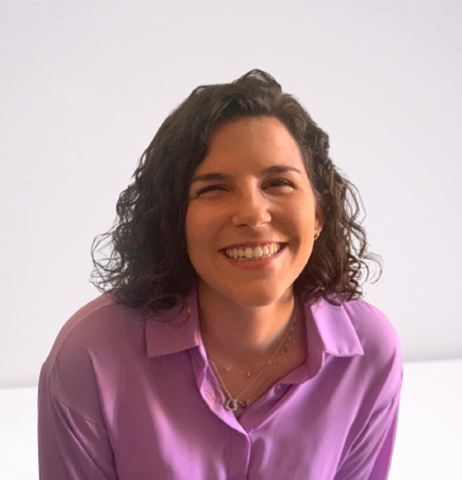
Marchiori Manerba
Phd Student
University of Pisa
R.LINE 1 ▪ 2 ▪ 5
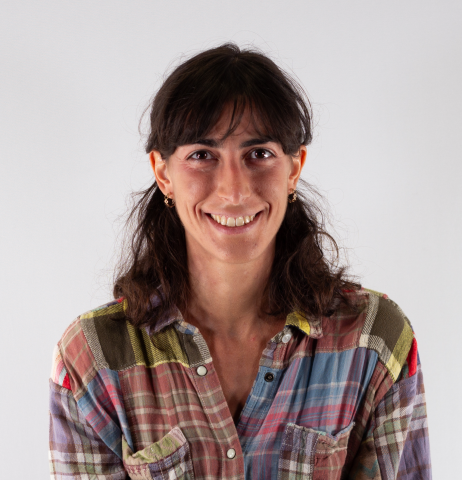
Punzi
Phd Student
Scuola Normale
R.LINE 1 ▪ 5
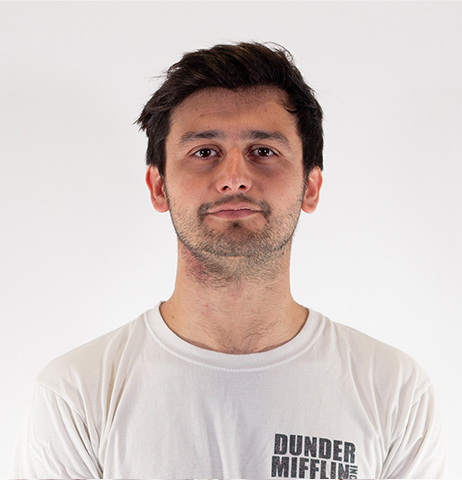
Pugnana
Researcher
Scuola Normale
R.LINE 2
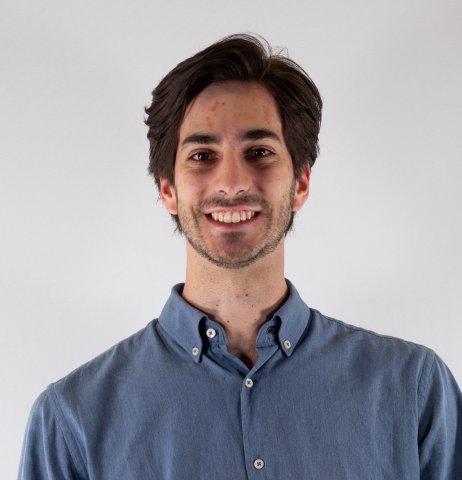
Lage De Sousa Leitão
Phd Student
Scuola Normale
R.LINE 1